In a highly competitive financial sector and with ever-evolving client demands, hyper-personalization is now a must to provide more relatable banking products. Tailoring banking services to meet the audience’s preferences and enhance their customer journey requires proactive effort. Addressing individual needs involves using the right tools, yet choosing the ideal software is challenging due to the variety of solutions available in the rapidly changing technological landscape.
The multitude of AI-powered solutions presented at industry events and discussed in business publications can make one’s head spin. Therefore, to navigate the complex world of artificial intelligence and natural language processing, we’ve brought machine learning knowledge closer, enabling readers to choose the best solution for their companies and leverage banking client data effectively.
Outlining the main benefits, various applications, example business scenarios, tech background, and brief development history might help determine if a given solution will indeed meet your financial company’s current data challenges. Let’s explore the capabilities of advanced machine learning models and find out why hyper-personalization, using real-time behavioral data and advanced algorithms for a much more personalized customer experience, is the next big thing in finance.
Banking customer data representation
Every financial institution stores a vast amount of unstructured data from multiple sources, and leveraging it wisely can give a competitive edge. As the amount of data grows, so does its complexity, hence the need for solutions to store and process it efficiently. Machine learning plays a crucial role in enhancing our understanding of customer characteristics.
To correctly present complex banking data in a way that allows its use by artificial intelligence algorithms, it’s necessary to apply vector data representation. This is where vector databases can make a significant impact. With better representation of customer data, it’ll be easier to make strategic decisions on banking product development and client segmentation.
Understanding vector databases, how they work, and how they store data is valuable not only to analysts and ML engineers but also to anyone seeking to extract meaningful insights from customer information. Our eBook focuses on issues related to a modern approach to banking customer data representation.
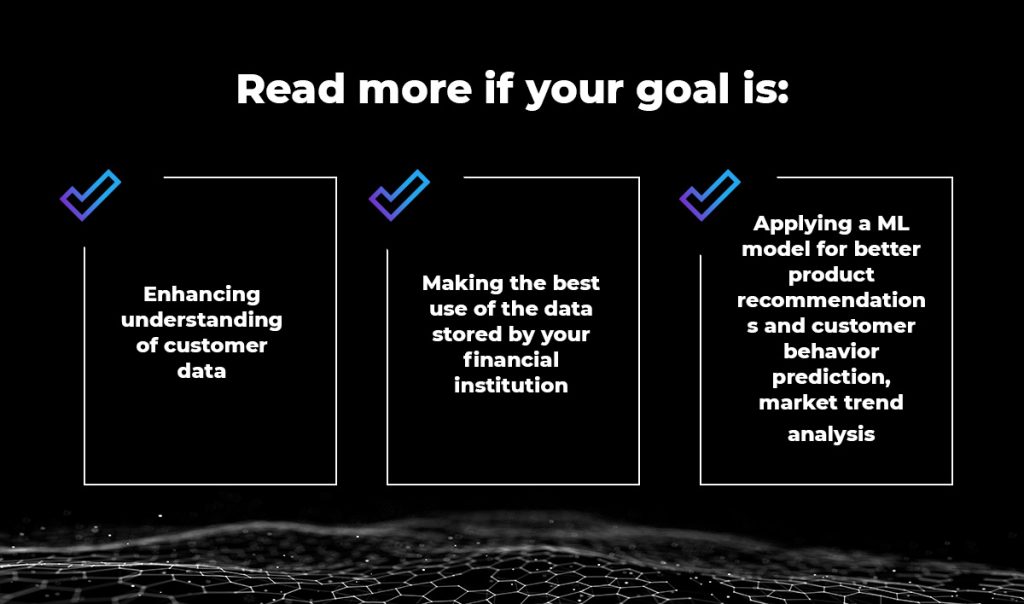
Maximizing the value of transactional data
Transactional data is one of the most valuable yet often underestimated sources of customer information. As the volume of data increases, the challenge arises: data is collected but not properly utilized in business processes, leading to its potential value not being fully leveraged.
Still, the primary tool used for transactional analysis is static analysis, which deeply examines transaction aspects like type, amount, recipient, description, and date.
The issue with transactional data analysis lies in the difficulty of automatically interpreting key attributes, such as recipient name or description, which reveal the customer’s true purchase intent. To maximize the potential of transactional data, additional attributes must be included using machine learning models. Our eBook covers the tech-related aspects of unlocking the full value of transactional data, proving that it offers valuable insights into customer preferences, enabling behavior prediction and market trend recognition.
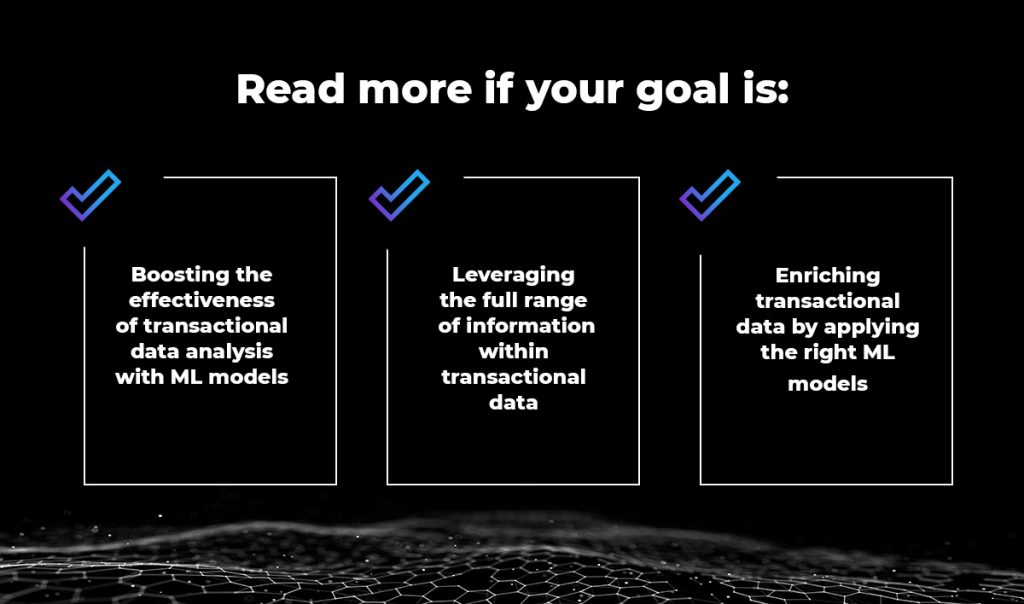
Counteracting the degradation of ML models over time – detecting concept drift and data drift
Research by MIT, Harvard, the University of Monterrey, and Cambridge found that 91% of machine learning models degrade over time. This indicates that developing a machine learning model is just the first step. Once deployed in a production environment, it poses a significant reliability challenge that requires continuous monitoring.
Data drift and concept drift are key factors to consider while maintaining the long-term accuracy of machine learning models. Data drift, interpreted as changes in the input data patterns and relationships that the ML model has learned during its training, might cause a decline in the performance of production models as time passes. An ML model may still work well with its static training data; however, the learned patterns might become outdated in new reality.
On the other hand, concept drift is defined as a change in the relationship between input data and target over time. Models trained on historical data may lose accuracy due to evolving correlations between input and output. Understanding concept drift and data drift is crucial for detecting and mitigating them. Our comprehensive eBook covers their causes and proactive measures to counteract them.
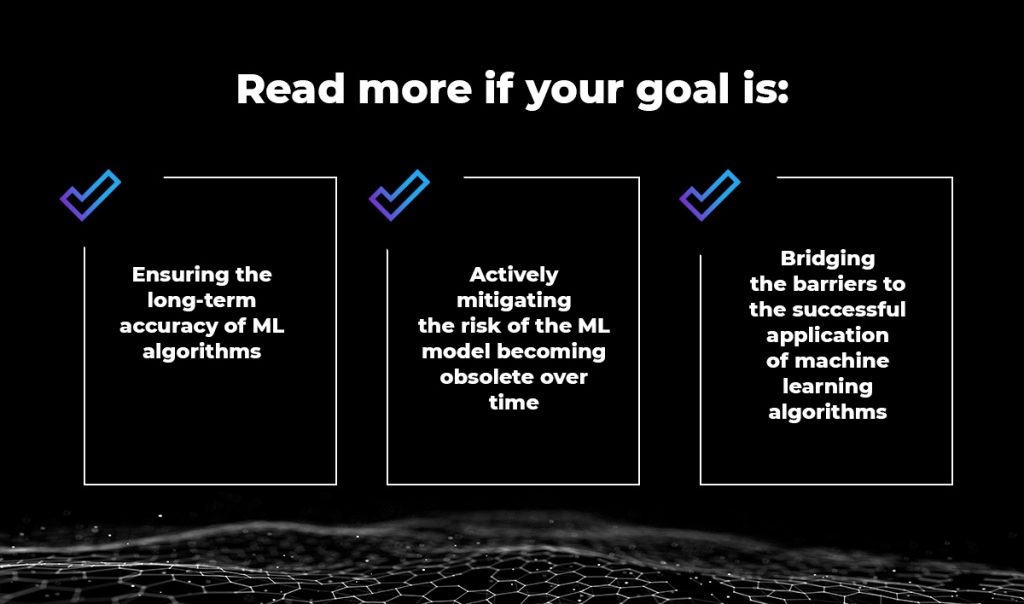
NLP and Generative AI changing the financial landscape
Generative Pre-trained Transformers (GPTs) have captured the entire business and technology world. No wonder – after all, they offer unparalleled capabilities when it comes to generating text outputs that accurately reflect human language. GPT models are widely used in banking and finance, automating everyday support tasks and providing faster, personalized customer assistance.
To fully understand the potential of Natural Language Processing (NLP), it’s worth taking a step back and exploring its fascinating history, which led to current technological advancements. By tracing the consecutive stages of Large Language Models (LLMs) development – we gain insight into the evolving role and objectives of NLP engineers, highlighting the field’s dynamic nature.
In our latest eBook we investigate how large language models (LLMs) have changed the approach to NLP, leading to the development of tools such as ChatGPT, Gemini, and GitHub Copilot. It also provides a detailed explanation of how GPT models work in practice.
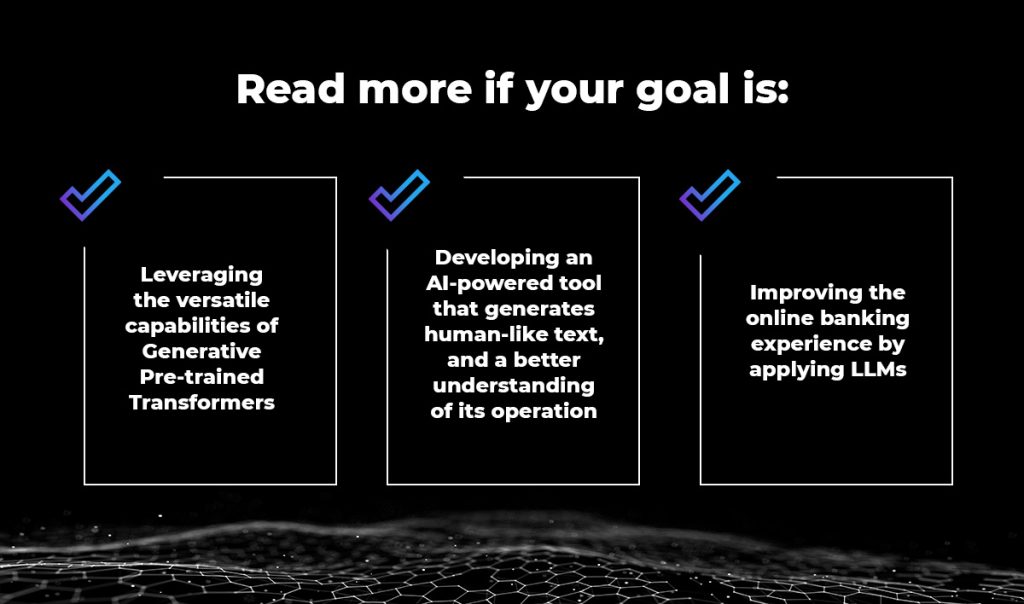
Acknowledgments go to the entire FTS Phoenix team at Ailleron, especially the authors of these eBooks: Miłosz Hańczyk, Izabela Czech, Łukasz Miętka, Miłosz Zemanek for their in-depth analysis and eagerness to share expert knowledge, and to Jakub Porzycki, Machine Learning Team Leader, for his mentoring and ongoing support.