Every new customer, every transaction and every message sent – these are the sources of huge amounts of data that are growing in banks every day. Although they seem overwhelming, their proper use can contribute to a significant development of the company. And even without taking advantage of their unseen capabilities, it’s important to know that storing such data is a growing challenge. See what big data in banking and financial services looks like, what opportunities it offers, and what challenges it poses.
What is Big Data in banking?
As the name suggests, big data is extensive (and even massive) amounts of data that flow into the bank every day. They relate in particular to transactions carried out by customers (e.g., their descriptions or amounts for which they are paying), or actions taken by them (decisions on a credit, loan, etc.). Customer communication also provides new data – phone calls with customer service, e-mails sent, and even conversations with chatbots.
These are typical examples that come to mind when we think about the source of data in a bank. However, this is just the tip of the iceberg. In each bank, you can find data on customer behavior in electronic channels, information on marketing activities, and even customer data from partner sources. The list could go on and on.
Moreover, such data is created constantly – with every second, there is more and more of it. For this reason, it’s difficult to control, let alone analyze in more detail. Therefore, many institutions decide only to store the data. However, in order not to waste this huge potential, you can rely on modern data analysis.
What is Big Data analytics in banking?
No one would dare to start manually analyzing trillions of bytes stored in a database. Unfortunately, the human mind is unable to cope with such amounts of non-uniform and inconsistent information. For this reason, artificial intelligence is increasingly used to analyze big data in the finance industry. Although it resembles the human mind with its actions, it is able to process several times more data at the same time compared to a human. Therefore, it is great at spotting what is invisible at first glance in data sets. Let’s now look at the application of artificial intelligence to analyze big data in financial services.
Big Data use cases in banking
As we have already seen, data in banks come from many sources – they refer to customers, transactions, and marketing activities. Thanks to this, big data analysis can contribute to the development of institutions in various fields. Here are some of the most interesting use cases of big data analytics in banking.
A personalized consumer experience
Customer satisfaction comes from looking after them. This can be manifested by providing your customers with experiences tailored to their preferences – you propose offers that will interest them most and adjust the way of providing services to their preferred form of communication. As a result, your customer will feel like he or she is a valuable customer to your bank.
But how do we gather information about individual customers from the collected data and use it for efficient service? The Customer Data Platform works great for this task – it’s a tool that creates general customer profiles based on data from many sources. With this solution, you can quickly see a person’s style of action – what he/she needs, how much time the person spends making a decision and what makes him/her decide on a specific option. This way, you can provide better customer service and streamline marketing activities.
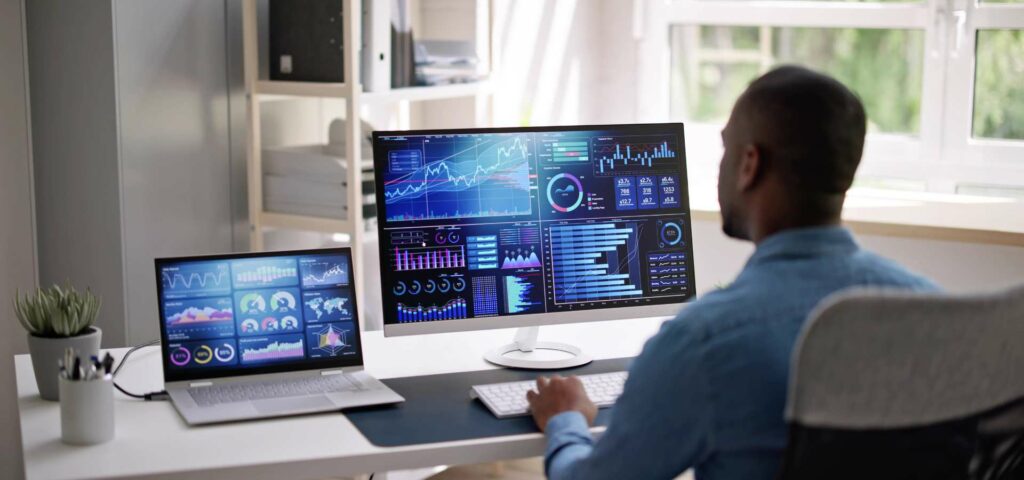
User Segmentation
The Customer Data Platform will also help with broader marketing activities. With CDP, you can divide (i.e., segment) customers into several groups based on their purchasing tendencies or propensity to make specific decisions. By doing so, you can easily tailor ads to a particular group of recipients and target the ones most likely interested in the bank’s offer. This is a great way to save money in marketing. CDP will help you reduce the funds you spend on misplaced ads and speed up the ad creation process with valuable tips on customer characteristics.
Optimization and automation of processes
Artificial intelligence is taking on more and more tasks performed by humans. Especially monotonous and repetitive tasks can be done incomparably faster by AI. (Machine Learning for Finance, Banking and FinTech – Cloud Machine Learning Services | Ailleron). It’s also easy to find such tasks in the financial sector – for example, an analysis of incoming documentation, recurring payments, or checking the correctness of completed documents.
Thanks to the large amounts of data collected by the institution, it’s possible to teach artificial intelligence to perform such activities successfully. This way, employees previously engaged in, for example, documentation analysis, can allocate the gained time for projects requiring creative activity, which cannot be dealt with by AI. What’s more, artificial intelligence usage eliminates errors that may occur as a result of employees’ fatigue due to the continuous performance of similar tasks.
Cybersecurity and risk management
Managing data security and financial resources should be a priority for every bank. Therefore, in such institutions, it’s worth using state-of-the-art methods to ensure the highest level of security. One of them is anti-fraud systems based on artificial intelligence and big data.
Anti-fraud systems allow you to quickly detect criminal transactions and take appropriate steps. However, those that do not use AI sometimes generate false-positive and false-negative errors. The first type of error occurs when a suspicious transaction is classified as neutral. Then, the criminals will not be stopped on time, which can lead to significant financial losses and the loss of the bank’s reputation. In contrast, false-negative errors occur when a neutral transaction is considered suspicious – as a result, the user’s account is blocked, leading to dissatisfaction with the bank’s services and even the loss of customers. However, by using AI and data collected by the company, you can gain a system that accurately classifies customer transactions and reduces the occurrence of these events.
Customer service
Big data in retail banking allows for a complete change in customer service. Everyone knows chatbots, which many companies are happy to use on their websites. However, the trick is to create a tool that will not only communicate with customers effectively at any time of the day but also provide them with valuable information.
Chatbots based on AI and big data can answer customer questions based on the entire history of their activities in the bank. They will quickly analyze the offers previously selected by the customer and propose new ones prepared by the bank. Consequently, the chatbot can suggest a suitable solution based on the source of the user’s problem. A human being would need much more time to do the same and may have difficulty recognizing all the nuances of cooperation with a given customer.
Chatbots can also serve a lot more customers than humans at the same time. Thus, the waiting time for the customer to solve a problem is significantly reduced, which positively impacts the bank’s perception. The analysis of big data also allows you to see recurring payments made by users and remind them of the transactions.
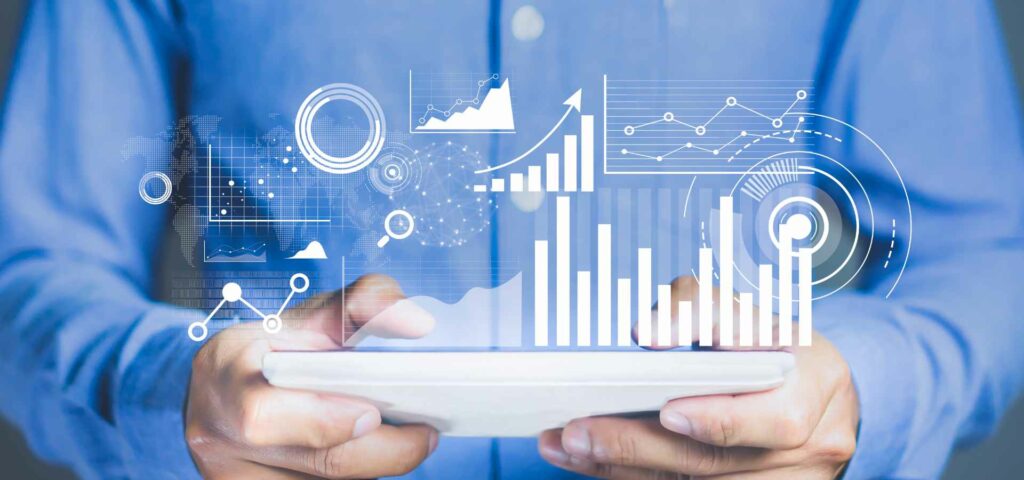
Classification of transactions
The combination of artificial intelligence and big data allows you to improve customer experience through the everyday use of a banking application. All thanks to an accurate analysis of the nature of the transactions made by a customer. AI, learning from large data sets, is able to properly assign a given payment by card or transfer to a specific category. This way, the customer can get acquainted with the structure of their expenses and see how they change over time. In addition, they can get tips on how to manage the budget more effectively.
Analysis of the customer’s creditworthiness
The bank primarily uses the customer’s credit history to assess whether a given customer can repay the loan he or she applies for. However, this is not the only source of information on the potential borrower’s habits – a lot about personal inclinations can also be learned from their social media profiles or the history of purchases.
Therefore, modern tools for creditworthiness analysis are increasingly using data widely available on the internet as well as profiles on Facebook or LinkedIn available to the bank. This way, in just a few minutes, banks are able to determine the credibility of a given customer, even if he or she doesn’t have a credit history yet. This is a great convenience both for the bank, as it can reduce the time spent on detailed analysis, and for the customer who might have a problem with receiving a loan from another institution.
Big data analytics in the banking industry – challenges
We have already seen that big data for banking can bring many benefits – it allows for a faster execution of tasks and the company’s development on many levels. Unfortunately, there are also some challenges related to big data for banks that need to be addressed at the right time.
Too much data
Big data is a general term for a broad data collection. When we mentioned them earlier, we considered only those that can be a valuable source of information and contribute to the development of a company. However, it must be remembered that among the trillions of data bytes there are also those that will not help in making business decisions or improving the customer experience. This is a normal phenomenon faced by all industries, not just the financial one.
How to deal with this problem, though? There are several possible ways – for example, designing a system that sifts irrelevant data from those that may be useful. On the other hand, you might think of creating a powerful artificial intelligence solution that would analyze all the available data, but it would certainly slow down its operation. Time will tell which solution turns out to be the most effective.
Problem with data security
It’s not just the analysis of huge amounts of data that poses a big challenge. It is equally vital to ensure they are protected from unauthorized access, especially when sensitive data on customer transactions and bank operations are involved. Leakage of such data could lead to significant financial losses as well as loss of reputation by the institution.
Therefore, it’s essential to ensure that the data storage is adequately secured. Artificial intelligence can also be used for this purpose – AI is able to analyze the user activity in the network on an ongoing basis and detect any suspicious behavior. Thanks to this, you can react quickly and thwart the hacker attack.
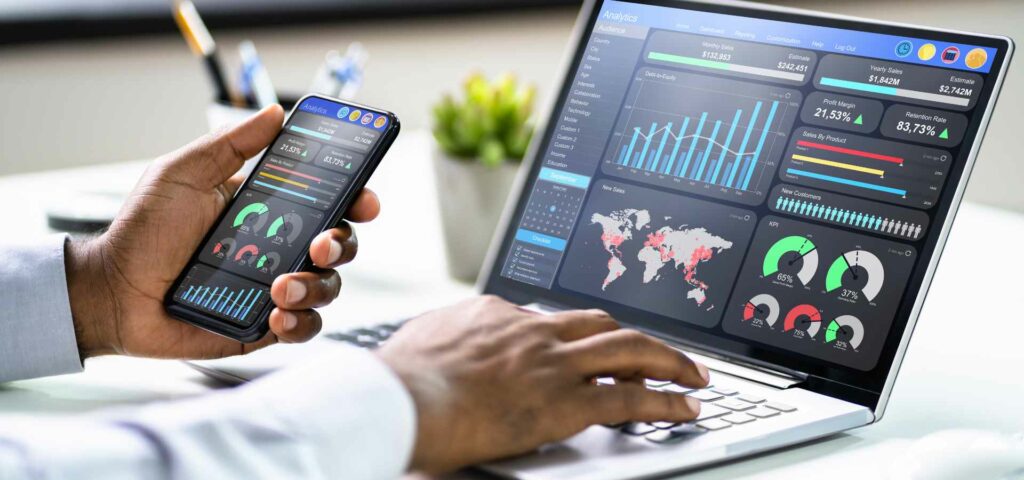
Compliance with data protection regulations
Big data stored by banks primarily consists of their customers’ personal data. Information of this nature should be handled with the utmost care. In addition, its protection is ensured by appropriate regulations. All European Union countries apply the General Data Protection Regulation (GDPR), which requires, among others, implementing data protection measures during the design phase and the default data protection. For this reason, big data processing must involve the development and introduction of new systems for the management of personal data. It may also be necessary to seek the support of a lawyer familiar with the GDPR.
Modern big data banking with Ailleron
More satisfied customers, faster bank operation, complete security of data storage and financial resources – these are just some of the possibilities offered by the use of big data for financial services. Do you think this is a good way to grow your bank? If so, take a look at the proposals from Ailleron!
Use big data with artificial intelligence
At Ailleron, we know that each bank has different needs and expectations regarding new solutions. That’s why we have prepared the Ailleron AI Banking module, which you can fully adapt to the requirements of your bank, choosing from the available ready-to-use elements. Do you want to analyze your customers’ past activity? Or maybe your bank would do well to automate repeated actions? Do you need support when making key decisions? Ailleron AI Banking module based on artificial intelligence and big data analysis will accomplish all these tasks!
Make the use of your bank services a pure pleasure
Having delighted customers is the key to any bank’s success . It can be achieved by providing users with a satisfying experience. Ailleron Customer Data Platform (CDP), a system that collects data from all available sources and creates a profile of each customer based on them, will work well for this purpose. A personalized way of communication, properly selected offers, and appreciation of regular customers – these are the possibilities offered by using CDP in the bank’s activity. Thanks to the analysis of endless amounts of data, it’s possible to see patterns in user activities and adapt future actions to them. In addition, using CDP gives you more control over the security of data storage and reduces the risk of leakage.
Don’t waste the potential of your data – use it for the unique development of your bank!
FAQ:
- How to use big data in banking?
Big data analytics in banking allows, among others, to improve customer experience, speed up the bank’s work and prevent criminal activities. Using large amounts of data will enable us to see trends invisible on smaller data samples and apply new information to the company’s growth.
- How can big data improve the bank’s operations?
Artificial intelligence based on big data can primarily automate repetitive and monotonous processes, like documentation analysis or recurring payments. In addition, big data helps to determine customers’ creditworthiness or to make the best decisions based on an advanced and complex analysis.
- What are the challenges of big data in banking?
First of all, big data, as the name suggests, is getting really big. As a result, it becomes more and more difficult to analyze such huge amounts of data, so over time, systems will be needed to separate valuable data from those which are less applicable. Also, a key issue is to ensure safety of the stored data.