About the client
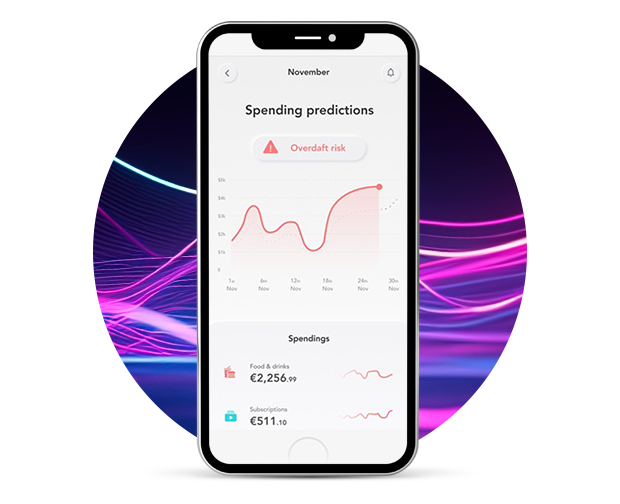
Personal finance management (PFM) software integrates seamlessly with banking systems, helping users manage their funds efficiently.
Our client, a cooperative bank from Poland, offered a form of PFM service for private customers as a part of the mobile banking app. However, PFM wasn’t sufficient for the customers’ needs:
- The rule-based logic unsuccessfully classified 3-5 out of 10 customer transactions
- The output data presented to end-customers required their interpretation and weren’t self-evident.
The cooperative bank asked Ailleron to improve the PFM experience and allow the customers to understand better and manage of their financial lives effectively.
Our experts designed and implemented AI-powered Ailleron Transaction Classifier (ATC).
The solution uses Machine Learning (ML) Models to support the bank’s customers in budget management.
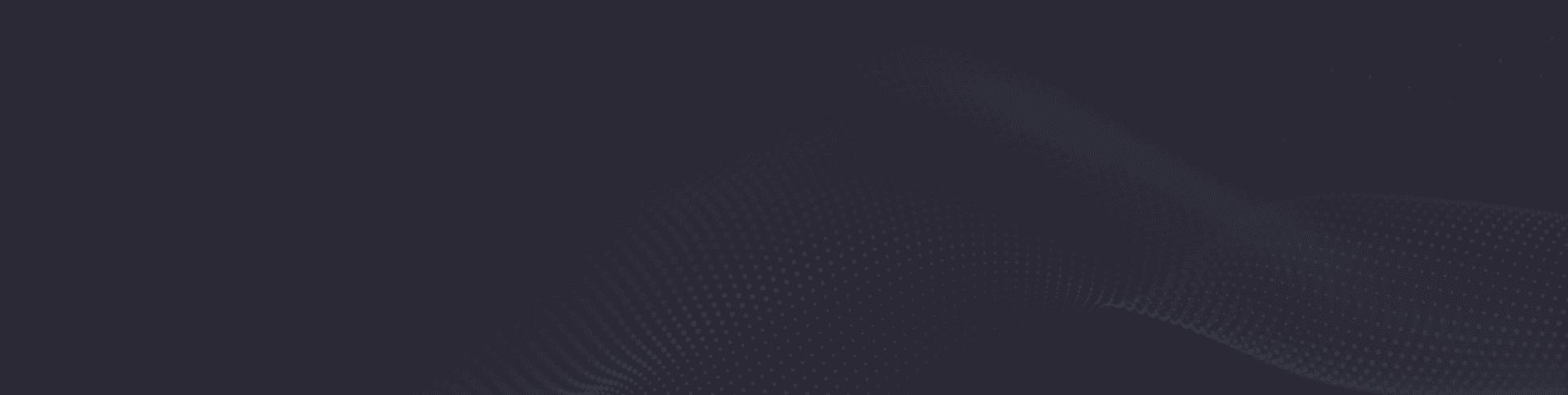
Solution:
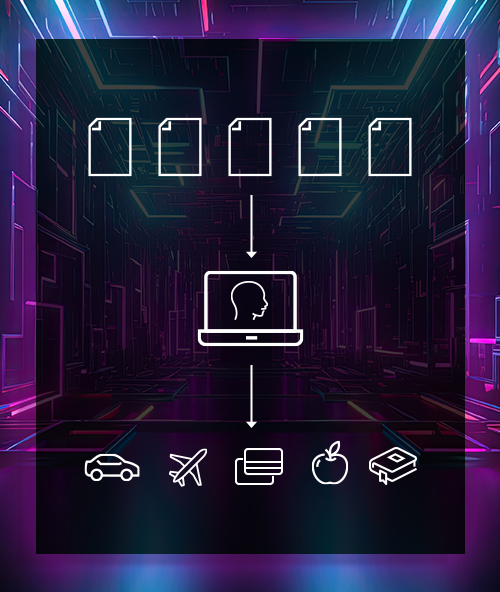
Our goal was to increase engagement among mobile banking app users by presenting customer transactional data conveniently. The project will bring significant short-term business benefits to the cooperative bank, including:
- increased mobile app engagement with a boost in logins and enhanced in-app activity after introducing new features,
- strengthened customer loyalty and trust by prioritizing their needs.
From a business perspective, structured customer spendings data are useful for:
- advanced analytical purposes – better customer segmentations and product recommendations
- enriching customer data
- building event-driven notifications for customers based on the history of their transactions
Implementation of ATC also allows for enrichments in customer segmentation.
Understanding resemblances in spending patterns enables identifying groups of similar customers accurately.
List of deliverables for the bank during the Ailleron Transaction Classifier project
- ML model as a microservice was prepared for implementation on the bank’s infrastructure
- Integrations with
- customer’s transactions sourced within bank systems
- mobile banking app
- Event-driven architecture for delivering real-time categorizations
- Data streaming platform based on Apache Kafka for gathering customer transactions and delivering to transactions classifier
- No-SQL data base –MongoB
- Tools responsible for monitoring, tracing, security, and log management:
Graphana, Proetheus, ISTIO, Zipkin, Flunent ID etc. - UX/UI to blend the new personal finance management (PFM) features into banks’ existing mobile application.
Key results of the project – top-notch accuracy
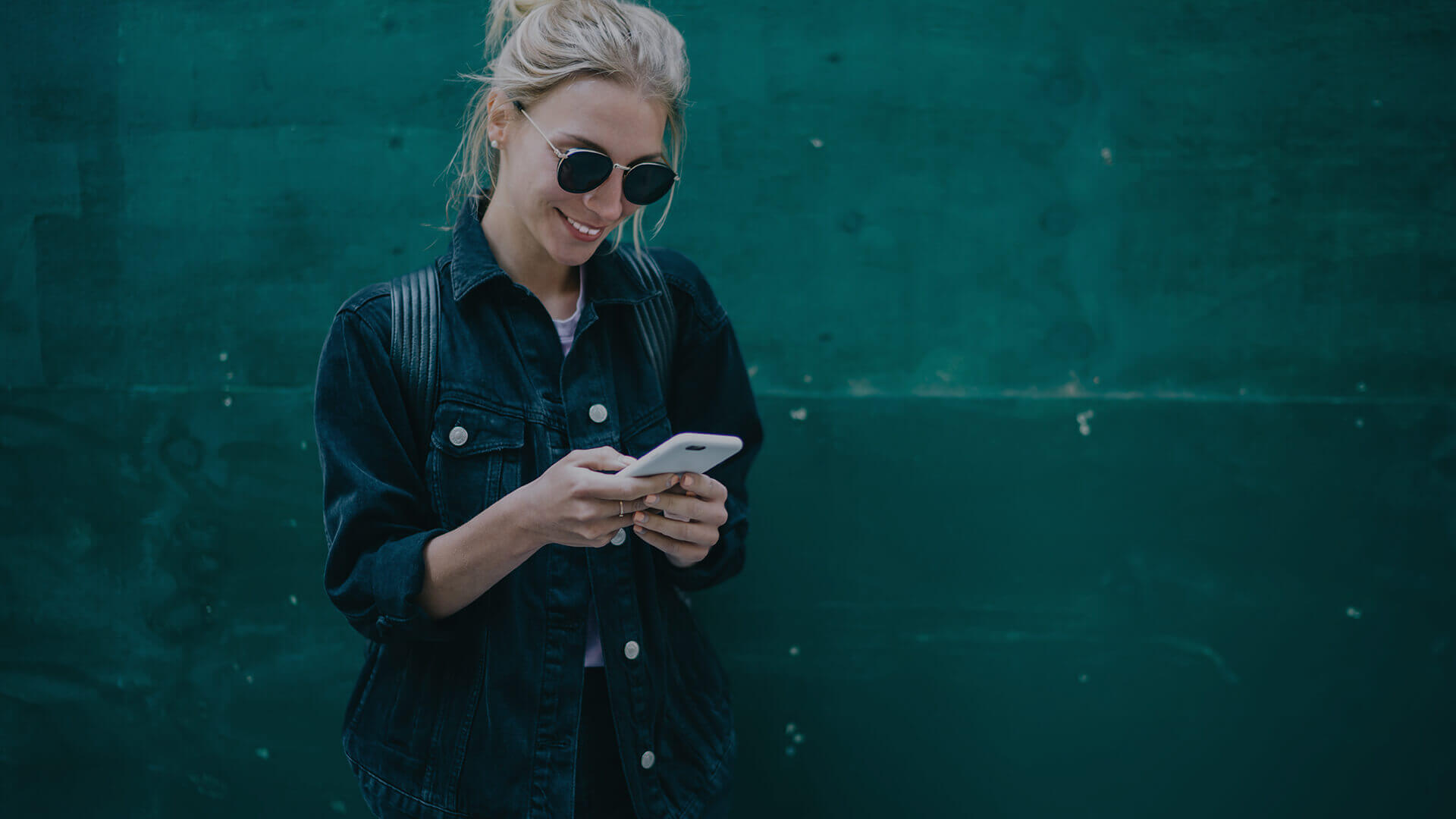
ATC divides customer transactions into 13 groups
- 11 groups for spending
- 2 groups of incoming transactions
The accuracy of the ML-model powering ATC is
- 92%, which means 92% of customer transactions are properly qualified (for transactions with or without MCC)
- 98% for the value of correctly qualified customer transactions, which means minor mistakes could occur for low-amount transactions.
We love data challenges! Let’s talk about how we can help your organization turn data into value.
Michał Walerowski
Business Unit Director AI/ML & Data Solutions
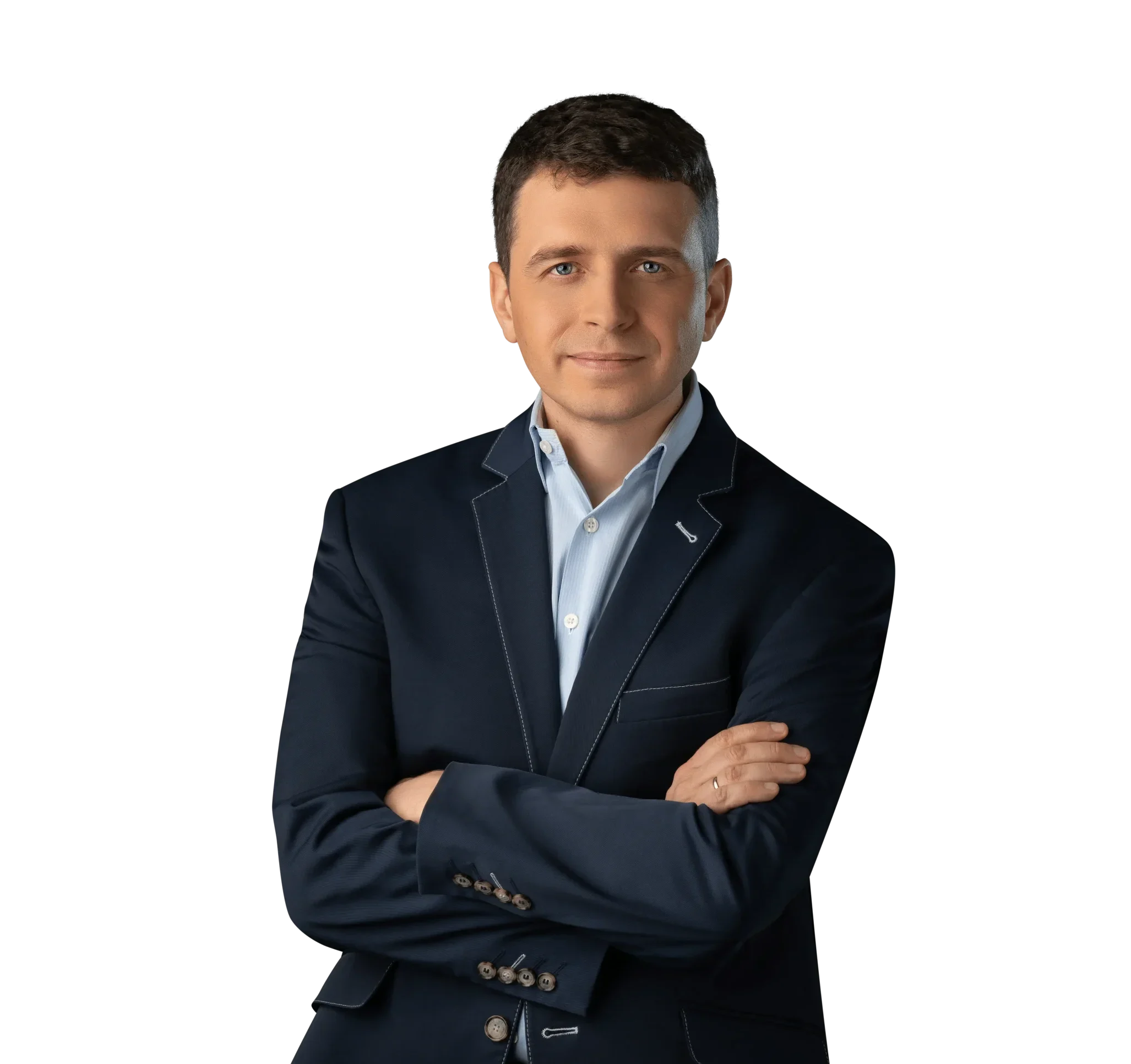
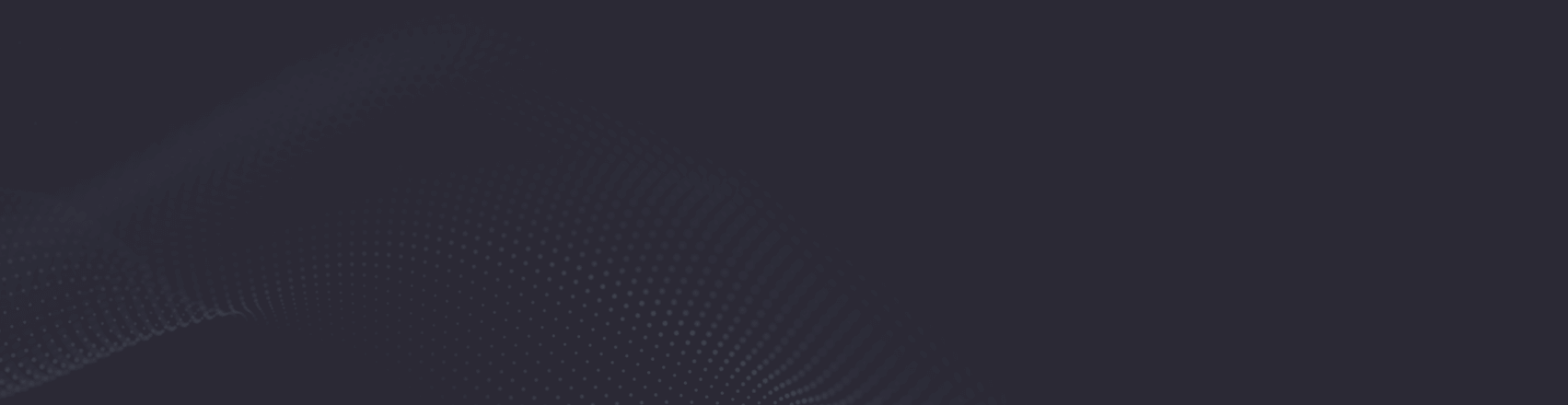
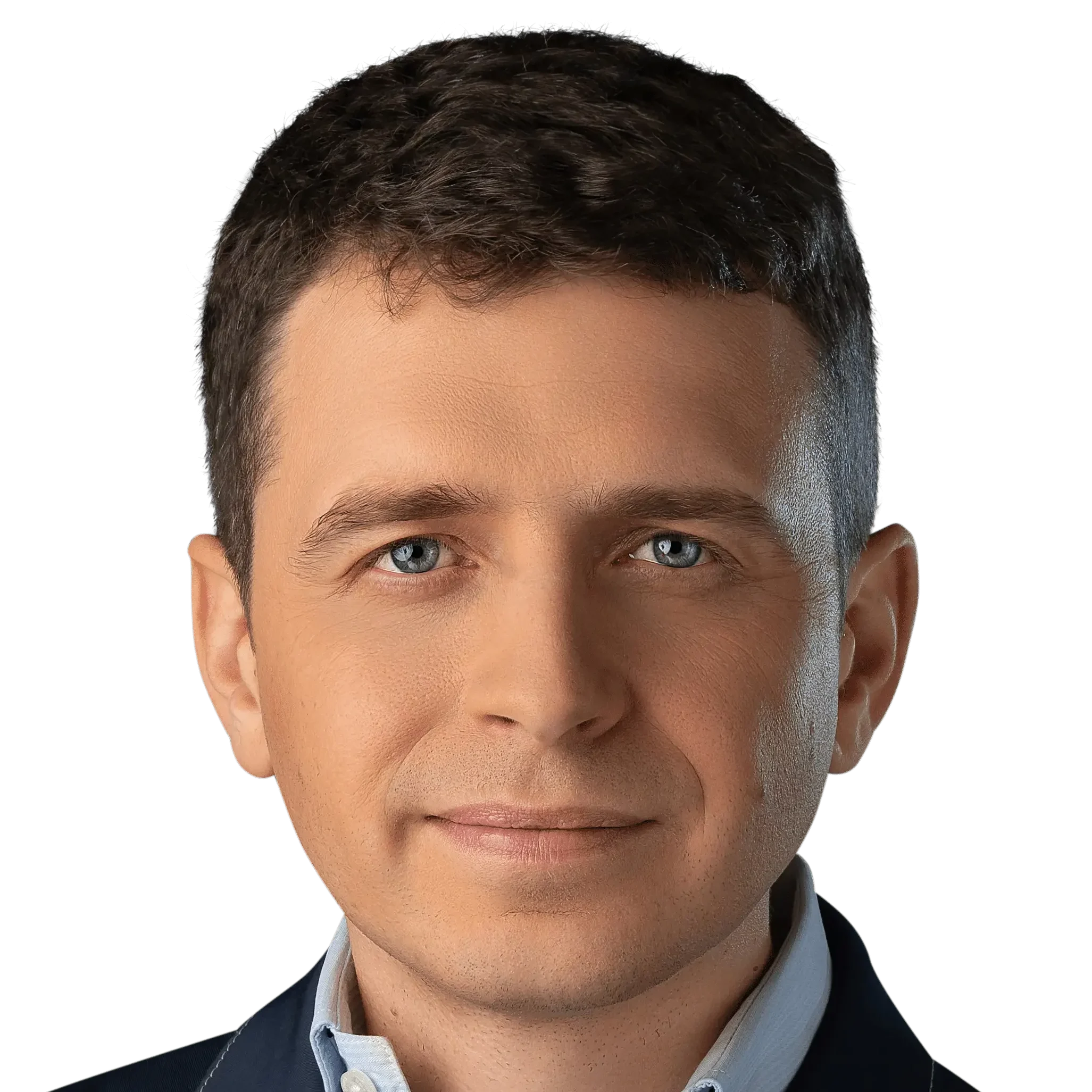
Let’s talk about your challenges with data